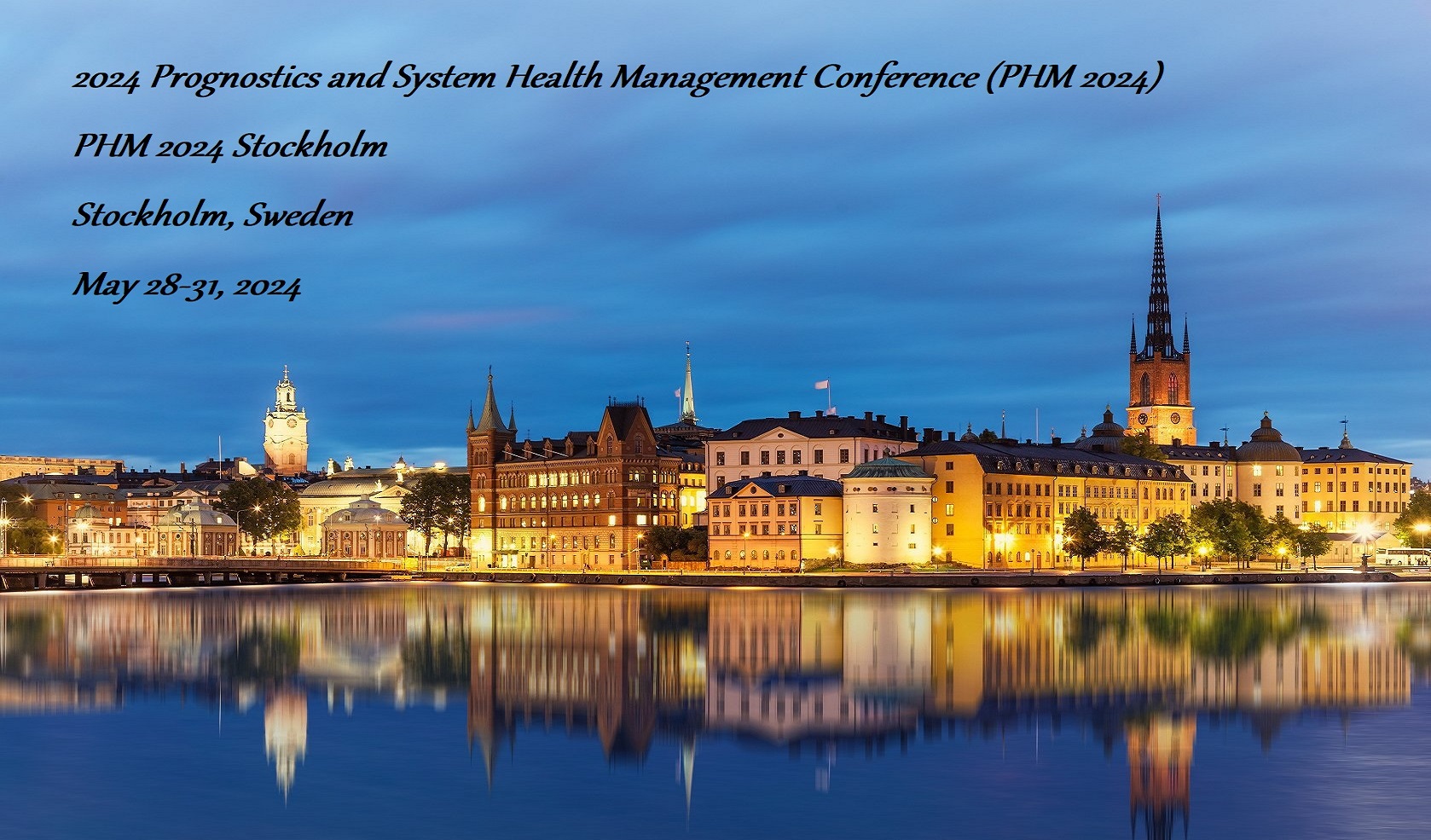
KEYNOTE I
Title: Prognostics and Health Management via Recurrent Expansion: Exploring Deeper Representations than Deep Learning
Keynote Speaker:
Dr. Mohamed Benbouzid, IEEE Fellow, IET Fellow, Professor, University of Brest, France
Abstract:
Machine learning applications for prognosis and health management usually face data unavailability, complexity, and drift due to the massive and rapid evolution of data volume,velocity, and variety (3V). Advances in deep learning have brought many improvements in this area, providing generative modelling, nonlinear abstractions, and adaptive learning to meet these challenges. Deep learning aims to learn from representations that provide a coherent abstraction of the original feature space, enabling it to be more meaningful and less complex. However, the data complexity associated with various distortions, such as higher noise levels, remains challenging to overcome. In this context, recurrent expansion algorithms have recently been introduced to explore deeper representations than ordinary deep networks, enabling even better feature mapping. In contrast to traditional deep learning, where abstracting inputs extract meaningful representations, recurrent expansion merges entire deep networks into one, allowing inputs, maps, and estimated targets to be explored as primary sources of learning. These three sources of information provide additional knowledge about their interactions in a deep network.
Furthermore, recurrent expansion provides the ability to investigate the estimated targets of multiple networks and learn significant features, improving its accuracy with each round.
This keynote will provide a general overview of recurrent expansion, its main learning rules, variants, and prospective developments in this context. Case studies on electromechanical systems will be provided to illustrate the effectiveness of recurrent expansion prognosis.
Speaker’s Biography:
Mohamed BENBOUZID is a Full Professor in electrical engineering, University of Brest, France. Prof. Benbouzid primary research interests and expertise include control of electric machines, variable-speed drives for traction, propulsion, and renewable energy applications, and fault diagnosis of electric machines.
Prof. Benbouzid is an IEEE Fellow and a Fellow of the IET. He is the Editor-in-Chief of the International Journal on Energy Conversion and the Applied Sciences (MDPI) Section on Electrical, Electronics and Communications Engineering. He is a Subject Editor for the IET Renewable Power Generation.
KEYNOTE II
Title: Research Progress of Prognostics and Health Management Technology for Power Conversion System
Keynote Speaker:
Dr. Y. Q. Chen, Deputy Chief Engineer of National Key Laboratory, The No.5 Electronics Research Institute of the Ministry of Industry and Information Technology, China
Abstract:
Power conversion system (PCS), such as electric drive and power supply, is the“Heart” of smart and electric equipment, such as aircraft, high-speed train and automobile and so on. And the reliability of the PCS is very important. There are some defects about the traditional reliability assurance methods based on pre-life prediction and post-failure analysis, such as high cost, low efficiency and poor accuracy. Prognostics and health management (PHM) technology is based on failure physics, which is used to predict and evaluate the reliability of a product in a real environment, and major accidents may be avoided. This report reviews the development status and quality reliability problems of PCS at first. Then, introduces the research progress of the physics of failure for the key devices of PCS. Next, expounds the research progress of the sensing and predication technology for the failure of PCS. At Last, shows the applications cases of PHM technology for the PCS.
Speaker’s Biography:
Yiqiang Chen is Full Research Fellow of the Science and Technology on Reliability Physics and Application of Electronic Component Laboratory, The No.5 Electronics Research Institute of the Ministry of Industry and Information Technology, China. His research interests include the failure mechanism and model of key devices, such as microwave devices, power devices and so on, prognostics and health management (PHM) of power conversion system (PCS), PHM of system on chip (SoC). He has successfully developed and verified eight kinds of chips for the failure monitoring and warning of SoC, and a series of instruments such as the semiconductor device quantitative evaluation system and power cycle test system, which provides a technical support for the reliability and security of electronic products. He has won the China Electronic Information Science and Technology Innovation Team Award, the Science and Technology Innovation Team Award of the Ministry of Industry and Information Technology, the working skills competition award of Guangdong Province, and the first, second and third prizes of provincial and ministerial level science and technology progress. He was selected as one of National Young Talents, the Outstanding Young Talents in Science and Technology Innovation of Guangdong Province, the Outstanding Natural Science Foundation of Guangdong Province, the Special Support Project, and the Pearl River Science and Technology Star and so on. He is the experts on national Science and Technology Achievements Evaluation Center, Ministry of Science and Technology of the People’s Republic of China, National Natural Science Foundation of China, Guangdong Province and Guangzhou City Science and technology project evaluation. He is responsible for more than 30 projects, such as national key R & D Program, National Natural Science Fund, Major International Cooperation Projects, high quality projects of MIIT and so on. As the first author or corresponding author, he has published more than 110 SCI papers in IEEE EDL, IEEE TED, IEEE TPE, IEEE TIE and so on. He has applied for about 100 international and domestic invention patents, of which 7 U.S. invention patents and 45 Chinese invention patents have been granted. He has co-published 3 books, 10 standards, and 5 sets of software.
KEYNOTE III
Title: Industrial Challenges and Current Research with the Green Energy Transition
Keynote Speakers:
Carl-Maikel Högström, Program Manager, Vattenfall R&D laboratory in Älvkarleby, Sweden
Ryad Zemouri, Data Scientist, Hydro-Québec, Canada
Abstract:
Power grids are changing rapidly, requiring a revision of their architecture, planning and operation to maintain stability and security in a context of increasing variability and uncertainty. This change requires an increase in the number of physical variables to be measured and monitored. In addition, the rapid expansion of renewable energy sources, increasing demand for electricity, and the need to improve grid reliability have necessitated the development of advanced technologies for managing power generation and transmission assets. As a result, the electric power sector is facing several operational challenges. In this presentation, we'll explore the industrial and technological context we face today in the energy sector. What are the challenges and current research with the green energy transition in terms of modeling, experimentation, and deep learning algorithms. Two industrial contexts will be illustrated: the North American context, presented by Hydro-Quebec, and the European context, presented by Vattenfall AB, R&D.
Speaker’s Biography:
Carl-Maikel Högström is Program Manager at Vattenfall R&D laboratory in Älvkarleby, Sweden and has over 24 years of experience from the hydropower industry. He holds a M. Sc. in Mechanical Engineering from Royal Institute of Technology (KTH) with major in fluid mechanics. He is leading several activities and research projects related to lifetime assessment and structural health monitoring a of hydroelectric turbines, generator, and auxiliary equipment. Ryad Zemouri received his engineering degree in electronics and control science from University of Tizi Ouzou (Mouloud Mammeri University) in Algeria, in 1998, a post-graduate diploma and a Ph.D. degree from the University of Franche-Comté in Besançon (France) in 2000 and 2003, respectively. In 2003, he joined the Conservatoire National des Arts et Métiers (CNAM) in Paris/France as an Associate Professor. In 2022 he joined the Research Center of Hydro-Québec (CRHQ) as a data scientist and PHM researcher. His research interests include machine learning applied for Prognosis and Health management of complex hydro-power generation assets.
|
|
|||||
|
![]() |
![]() |
||||
|
![]() |
![]() |