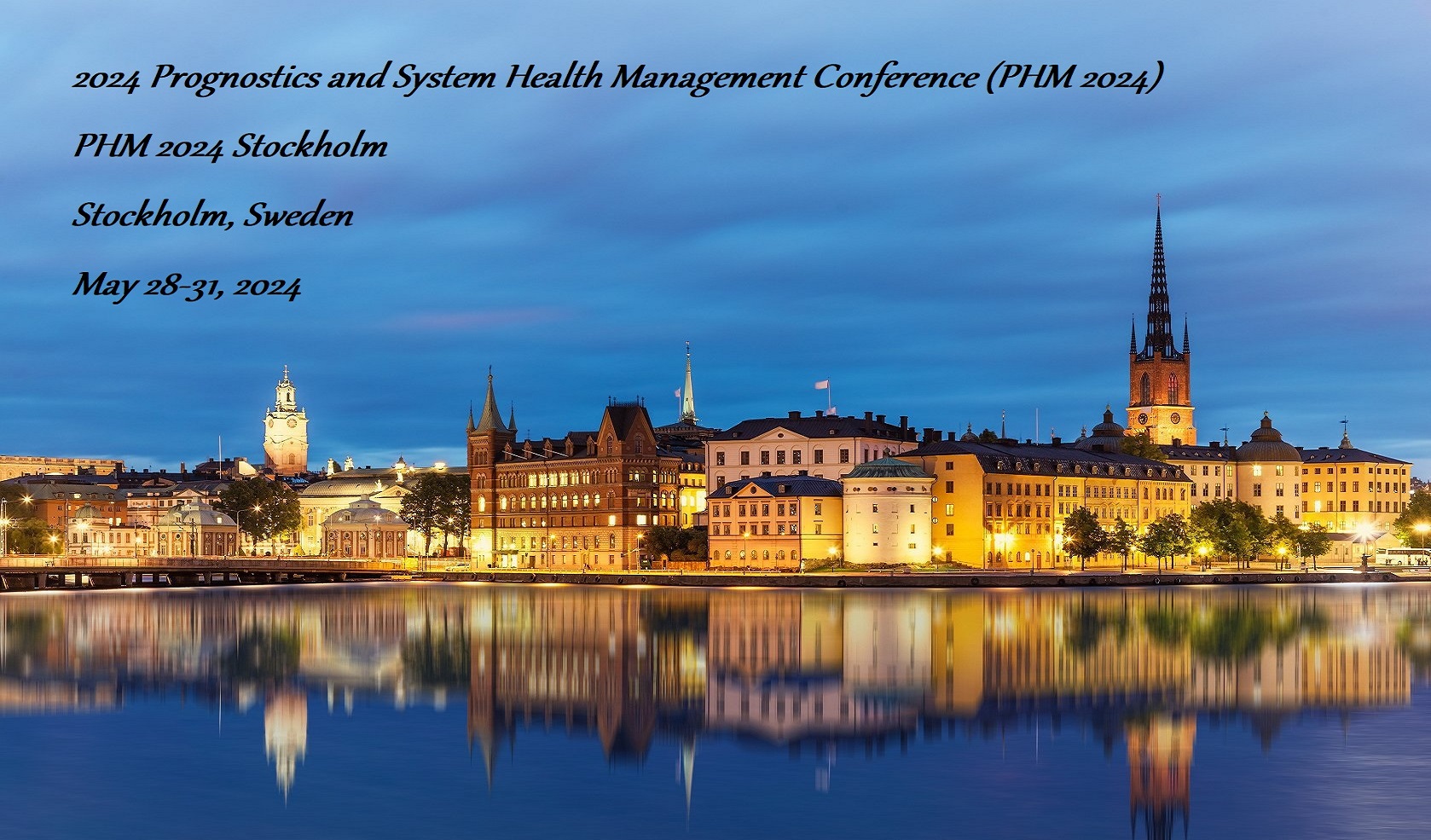
Special Paper Session: Graph Neural Networks for Prognosis and Health Management
Predictive maintenance (PdM) has consistently attracted the interest of the industrial community due to its significant potential for reducing maintenance costs while increasing equipment reliability. Current PdM research has focused much attention on fault perception (anomaly detection), whereas the processes of identifying the fault source and estimating its future evolution are more complex functions, influenced by many other factors. Most existing approaches have not been able to effectively manage existing knowledge to determine the cause-and-effect relationship between failures. Furthermore, the complete analysis of the correlation between identified failures and the corresponding root causes represents a major challenge for industry. Graph Neural Networks (GNN), associated with the concept of cognitive intelligence, represent an interesting avenue to explore. These methods may prove superior in semantic causal inference, heterogeneous association, and visual explanation (interpretation). In addition, these methods can achieve promising performance in reasoning tasks in a PdM process by revealing the dependency relationship between systems and/or equipment components. Recently, GNNs as the emerging neural networks have been widely used to model and analyze the data. However, there still lacks a guideline on leveraging GNNs for realizing intelligent fault diagnosis and prognostics.
We expect this special session to result in coherent and focused contributions on the topic of Graph Neural Networks for PHM. By carefully selecting complementary contributions from academia and industry, we expect this special session will be a forum to exchange knowledge between these two worlds. This special session dedicated to GNNs applied in the PHM will provide a platform for researchers and practitioners to exchange their ideas and findings, discuss the latest developments, and explore future directions in the field of the Graph Neural Networks approaches for Prognostics and Health Management.
For a better organization of this special session, please send a notification e-mail to the organizers as soon as you submit your contribution via the submission platform.
Organized by:
Ryad Zemouri (zemouri.ryad@hydroquebec.com) Research Center of Hydro-Quebec, Canada
Hung Pham (Pham.QuangHung2@hydroquebec.com) Research Center of Hydro-Quebec, Canada
![]() |
||||
![]() |
||||
|
![]() |
![]() |